Harnessing generative AI for enhanced scientific research in the energy, oil, and gas industry
In the realm of material science, particularly within the energy, oil, and gas sectors, generative AI (GenAI) is making significant strides, not just in hypothetical ‘moonshot’ applications as highlighted by McKinsey, but in practical, impactful ways.
When implemented correctly with a focus on cost-efficient domain expertise, GenAI can revolutionize the way scientific literature and corporate patents are summarized and analyzed, providing researchers with timely, actionable, and scalable scientific insights.
If the energy industry is to progress to a more sustainable future, where it can maximize the potential of green technologies and energy storage, leveraging the scientific research available will be key to the transition.
The current landscape
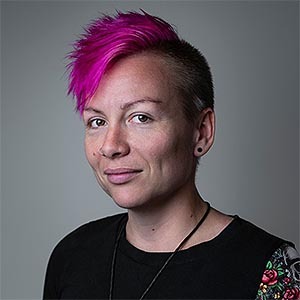
The scientific process entails evaluating most of the literature available, to assess the consensus of the research area in question. This is no different for researchers operating in energy, oil, and gas businesses, who are limited by the number of papers they can read at any given time.
Sifting through vast amounts of data and literature is a time-consuming task that can delay the application of findings to real-world problems. However, artificial intelligence (AI) has begun to streamline this process significantly.
By automating the review and summarization of countless scientific documents and patent documentation, GenAI can allow researchers to quickly grasp actionable key findings at scale and integrate them into their projects. This can range from improving safety on oil rigs, as is currently being done by Argonne National Labs, discovering new materials that can improve battery storage capacity or enhance the output of wind and wave turbines.
Streamlining discovery
One of the foremost benefits of GenAI is its ability to process and summarize large volumes of scientific literature rapidly. This capability is crucial in a field where new research papers, reports, and data are constantly being published.
GenAI tools use advanced natural language processing techniques to identify, extract, and present the most relevant information from these documents, effectively reducing the time researchers spend on literature review from weeks to mere hours.
Yet the benefits go much further than just time efficiencies. Beyond simple summarization, AI can aid researchers in spotting trends and patterns across extensive datasets that may be imperceivable to the human eye alone. We saw this last year when Google’s DeepMind discovered 2.2 million crystals, 380,000 of which were stable materials that could help power future technologies. Google cited the discovery as equivalent to 800 years of knowledge, an incomprehensible amount of intelligence for a human alone to process.
As the technology becomes increasingly democratized, it’s easy to see how similar scalable insights can be applied to the energy sector. In the exploration of new chemical pathways for energy production, AI algorithms can predict the viability of new compounds or techniques by correlating data from diverse sources. This not only accelerates the pace of innovation but also reduces the likelihood of costly research dead-ends.
Cost-effective and practical solutions
Much of the discussion around large language models (LLMs) is currently focused on their huge requirements for power and hardware, which makes both deploying and running them incredibly expensive.
Yet there are alternative ways. Implementing the appropriate language model does not always require a huge amount of investment in time and resources. Many AI models have much smaller parameter counts and are trained with specific domain expertise in mind, making them much easier to integrate with current technological infrastructures, so they are both cost-effective and scalable. This ease of integration means that even smaller research teams can leverage AI tools without the need for significant capital investment, democratizing access to cutting-edge technology.
Being tailored to specific domains also means these models can prioritize accuracy and reliability, unlike LLMs that are trained on broad corpora of data from across the internet. The power of LLMs can be combined with the cost efficiency of smaller models, and with a variety of techniques can prioritize facts and prevent hallucinations.
Researchers need to be able to trust the summarizations they are reading are valid representations of the literature as a whole. There are techniques for cross referencing factuality, such as data citation and knowledge graphs, that are not available on popular LLMs alone.
The future impact
As the technology evolves, the potential applications of GenAI in the energy, oil, and gas industry will expand. Future developments could include more sophisticated predictive models for resource exploration, automated safety monitoring systems, and real-time environmental impact assessments. The key to these advancements lies in the continuous refinement of AI algorithms and the integration of ever-more complex datasets.
While McKinsey highlights the potential for revolutionary applications of AI in the energy sector, the practical, current impacts of GenAI are both profound and transformative. As these tools become more integrated into everyday research activities, their ability to deliver timely, actionable insights will undoubtedly make them indispensable to researchers within the sector. The ongoing collaboration between AI experts and industry researchers will be crucial in realizing the full potential of GenAI, ensuring that the energy, oil, and gas sectors can meet future challenges with the best tools at their disposal.
By Anita Schjøll Abildgaard
For a list of the sources used in this article, please contact the editor.
Anita Schjøll Abildgaard is CEO and Co-founder of Iris.ai, one of the world’s leading start-ups in the research and development of artificial intelligence (AI) technologies. Founded in 2015, the start-up offers an award-winning AI engine for scientific text understanding. The company uses natural language processing/machine learning to review massive collections of research papers or patents: find the right documents, extract all their key data, or identify the most precise pieces of knowledge. Iris.ai collaborates both with innovation-oriented universities and corporate customers and contributes to many joint research projects fostering open science and innovation.